Data Science Certification Training Course Using R
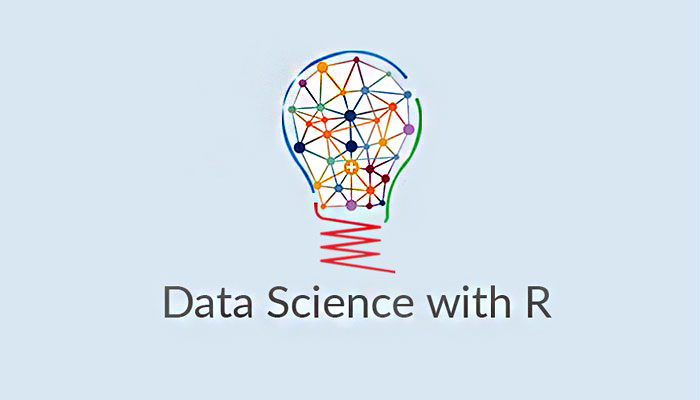
Data science with r from CertOcean helps gain skills using R in
machine learning algorithms such as K- Means Clustering, Decision Trees,
Random Forest, and Naive Bayes. This data science learning includes a
quantitative understanding of statistics, time series, text mining, and
an introduction to deep learning have. You will be integrating real-life
use cases for advertising, healthcare, social media, aviation, and HR
in this data science course.
WHY SHOULD ONE LEARN DATA SCIENCE?
* The number of job offerings for data science and analytics will accelerate by nearly 364,000 positions by 2020 - Forbes.
* For a data scientist, the average annual salary is $120k, according to Glassdoor.
* Data processing firms will see $430 billion in efficiency improvements over their opponents who do not examine data by 2020.
Course Curriculum
Objective learning: In this tutorial, get an orientation to data science and data science with r and further
see how Data Science helps explore massive and unstructured data with various techniques.
Topics Covered:
* What exactly is Data Science?
* What is associated with Data Science?
* The Data Science Period
* Intelligence with business vs. data science for
* Data Science's life cycle
* Data Science Techniques
* Big Data and Hadoop Development
* Demonstration to R
* Spark Overview
* Machine Learning Overview
this tutorial.
Topics Covered:
* What is an Inference from Statistics?
* The Statistics Conceptual frameworks
* Centers' Measures
* Spread Measures
* The probability
* Regular Distribution
* Binary Division
standardized manner, analyze the data, and graphically view it.
Topics Covered:
* Pipeline for Data Analysis
* What is Data Mining?
* Data Types
* Raw Data and Processed Data
* Wrangling of Data
* Review of Exploratory Data
* Data Visualization
* Practical Performed:
* Loading various dataset forms into R
* Coordinating the data
* Preparing the graphs
apply Supervised Learning Algorithms in the multiple areas of Machine Learning.
Topics Covered:
* Machine Learning, what is it?
* Usage of Machine Learning-Cases
* Process for Machine Learning Flow
* Categories for Machine Learning
* Linear Regression and Logistic Regression: Supervised Learning Algorithm Practical Performed:
* Implementing the model of Linear Regression in R
* Implementing the model of Logistic Regression in R
Topics Covered:
* What are the cases for classification and its use?
* What's the Tree of Decision?
* Decision Tree Induction Algorithm
* Build a Perfect Tree of Decision
* Matrix for Ambiguity
* What is the exact meaning of Random forest?
* What is the exact meaning of Naïve Bayes?
* Support Vector Machine: Efficient method of classification.
Practical Performed:
* Implementing the model Decision Tree in R
* Linear Random Forest in R Application
* Implementing the model of Naive Bayes in R
* Support Vector Machine integrated into R
analysis varieties used to examine/analyze the data.
Topics Covered:
* What is clustering & its use cases?
* What is clustering in K-means?
* How exactly does the algorithm for K-means execute?
* How can optimal clustering be gained?
* What is the meaning of Clustering in C-means?
* What is the meaning of Clustering in Hierarchy?
* How does Hierarchical Clustering work perfectly?
Practical Performed:
* Executing Hierarchical, C-Means, and K-Means clustering technique
Recommender Engines.
Topics Covered:
* What are association laws & cases of use?
* What is the Recommendation Engine, and does it work?
* Recommendation styles
* Recommendation based on customer
* Recommendation based on products
* Difference: Advice depending on the customer and object
* Use cases of recommendation
Practical Performed:
* Implementing Rules of Association in R
* Construction in R of a recommendation engine
Section of various algorithms such as TF-IDF and cosine Similarity.
Topics Covered:
* Text-mining principles
* Using cases
* Mining Algorithms for Text
* Quantification of Text
* Concept of TF-IDF
* Moving beyond TF-IDF
Practical Performed:
* Going to implement the solution of Bag of Words in R
* Going to implement Sentiment Analysis using R on Twitter Data.
data, Time Series models - Exponential Smoothing methods, and Time Series Forecasting ARIMA model.
Topics Covered:
* What is data from the Time Series?
* Variables from the Time Series
* Different Time Series data components
* Visualize the data for Time Series Analysis And optimization
* Implementing the ARIMA forecasting model
* Models of Exponential Smoothing.
* Detection of different time series examples based on the implementation of several exponential smoothing mechanisms
* Implement the corresponding model of ETS for forecasting
Practical Performed:
* Visualizing and formatting data from the Time Series
* Decomposed Time Series Data Graph Plotting
* Applying the Time Series Forecasting ARIMA and ETS model
* Forecasting for the period required.
Objective learning: This method teaches you to the terms of Reinforcement Learning and Deep Learning.
With the aid of Use Cases, we will clarify these definitions. You will address the Artificial Neural Network,
the Artificial Neural Network building blocks, and a few concepts for the Artificial Neural Network.
Topics Covered:
* Learning of Reinforcement method
* System Flow of Reinforcement Learning
* Reinforced Education Use cases
* In-depth learning of basic terms.
* Neuronal Biological Networks
* Understanding Neural Artificial Networks
* How ANN functions?
* Essential terminologies of ANN
Course Description
* CertOcean's data science course focuses on the basic methods of data science with r. To "concept to data analysis, unify statistics, and their related methods" with data, data science seems to be a "understand and analyze actual phenomena." Data Science Training uses methods and approaches derived from the sub-domains of machine learning, classification, cluster analysis, databases, data mining, and visualization from many fields within the diverse areas of mathematics, information science, statistics, and computer science.
* The data science course aims to gain visibility into Data Science's entire lifespan, explore and imagine various data sets, multiple machine learning algorithms, including K-Means Clustering, Decision Trees, Random Forest, and Naive Bayes.
The data science course delivers:
* In-depth understanding of the life span of data science with r and machine learning algorithms
* Comprehensive knowledge of different Data Transformation tools and methodologies
* The ability to execute Text Mining and Sentimental text data analyses and gain some insight into the aspects of data visualization and optimization
* Analyze multiple data forms using R
* Describe the Development Process of data science
* You will learn to deal with multiple data formats, such as XML, CSV, etc.
* Learn Data Transformation tools and techniques
* Discuss the methodology of data mining and its execution
* Data processing using R machine learning algorithms
* Collection of the movies
* Predicting real estate money
* Predicting diabetes problem
* Building up a recommendation engine for some grocery market
* Analysis of Twitter
* Forecasting of air passengers
Features
Live Instructor-led Classes
30 Hours of Online Live Classes. Weekend class: 10 sessions of 3 hours each. Weekday class: 15 sessions of 2 hours each.
Real-life Case Studies
Live project based on any of the selected use cases, involving the implementation of Data Science.
Lifetime Access
Where tutorials, quizzes, installation guides & class recordings are available, you will get lifetime access to LMS.
Special Support 24x7
We have a 24x7 lifetime digital support staff, via a ticket-based monitoring system, to answer all your technical questions.
Certification
Complete your final course project successfully, and CertOcean will certify you as a 'Data Science Expert' based on the project.
Discussion Forums
For our learners, we have a social forum that further enhances innovation through peer engagement and information sharing.
Frequently Asked Questions (FAQs):
* You can view the recorded sessions and attend another live batch, and thus, in this way, you'll never miss a class.